Sometimes, the tech buzzwords of the moment are used so freely when speaking to colleagues and customers and read daily in articles, on social media, and even in the mainstream news. Although terms such as AI, gen AI, edge computing, digital twins, IoT, and sustainability are familiar, their practical implementation is challenging. The challenges and obstacles are numerous and, at times, unique to specific use cases or organizations and depend on the maturity of the emerging technology.
Consider digital twins as an example; what are they, and what is all the hype surrounding them? The definition used by the Digital Twin Consortium describes a virtual representation of real-world entities and processes synchronized at a specified frequency and fidelity with the capability of transforming business by accelerating holistic understanding, optimal decision-making, and effective action. Digital twins use real-time and historical data to represent the past and present and simulate predicted futures. Furthermore, digital twins are motivated by outcomes, tailored use cases, powered by integration, built on data, guided by domain knowledge, and implemented in IT/OT systems.
Suppose we take a manufacturing plant as an example. Whether the equipment used is of new generation or legacy and has fixed function, general-purpose devices, or a combination, one thing is for sure: an overwhelming amount of data is produced. The data is a modern-day goldmine if extracted, processed, aggregated, and verified. Data allows digital twins to thrive, and its integrity is one of the most critical aspects of the technology. It is what helps ensure consistent, accurate, reliable results. In the future, IT and OT resources and infrastructure will need to converge further to standardize, transform, and apply data insights in manufacturing settings.
The accuracy of the data allows us to rapidly create physically precise, virtual 3D models and replicate real-world environments, from the factory floor to stores and cities.
Digital twins can be used to recreate the factory itself, allowing organizations to monitor and make changes in the digital environment to verify the impact of results before making changes on the factory floor. Manufacturers can also create an exact digital replica of their product and carry out true-to-world testing, allowing them to find and correct issues or errors and make optimizations before moving into production. Furthermore, digital twins create predictive models based on data points and their historical changes. They are measuring conditions against historical patterns and trends to identify anomalous behaviour, such as production line bottlenecks or potential safety and security breaches, right down to granular details, such as the temperature and vibration of a single appliance.
Considering today’s level of technological maturity, digital twins provide a range of benefits, including:
- Heightened visibility and transparency into assets and environments
- Reducing costs, time, and effort in changing production workflows
- Reducing material waste and delivering energy and other utility savings
- Sustainability
- Efficient acceleration of production times
- Reduced errors and issue resolution in pre-production phase
- Employing machine learning models that can understand and act in real-world situations.
So how are digital twins created?
Digital twins generally require purpose-built software on IoT edge servers that draw real-time data from sensors, appliances, and cameras. However, in most cases, organizations can start with their existing infrastructure and layer analytic tools to leverage the data already generated by installed equipment. Incrementally adding compute resources will help improve the accuracy of the digital twin over time. These considerations depend on what the organization is trying to achieve and its long-term goals. Technology is a strategic investment, so organizations should work with a reliable collaborator to plan for new use cases from infrastructure, resource, and security aspects.
The skill sets needed to leverage modern technology have evolved, and the workforce needs to evolve with that to obtain optimal results.
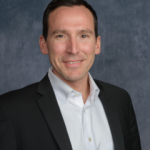
Director, Sales Intelligent Solutions,
OT + Emerging Accounts,
Arrow Electronics, Inc.