Modern ICs are enormously complicated. An average desktop computer chip, as of 2015, has over 1 billion transistors. The rules for what can and cannot be manufactured are also extremely complex. Common IC processes of 2015 have more than 500 rules. Furthermore, since the manufacturing process itself is not completely predictable, designers must account for its statistical nature. The complexity of modern IC design, as well as market pressure to produce designs rapidly, has led to the extensive use of automated design tools in the IC design process. In short, the design of an IC using EDA software is the design, test, and verification of the instructions that the IC is to carry out.
Cadence Design Systems, Inc announced the delivery of the Cadence Cerebrus Intelligent Chip Explorer, a new machine learning (ML) based tool that automates and scales digital chip design, enabling customers to efficiently achieve demanding chip design goals. The combination of Cerebrus and the Cadence RTL to-signoff flow offers advanced chip designers, CAD teams and IP developers the ability to improve engineering productivity by up to 10X versus a manual approach while also realizing up to a 20% better power, performance and area (PPA).
With the addition of Cerebrus to the broader digital product portfolio, Cadence offers the industry’s most advanced ML-enabled digital full flow, from synthesis through implementation and signoff. The new tool is cloud enabled on Amazon Web Services (AWS) and other leading cloud platforms and utilizes highly scalable compute resources to rapidly meet design requirements across a wide range of markets including consumer, hyperscale computing, 5G communications, automotive and mobile.
ELE Times correspondent Mayank Vashisht spoke With Venkat Thanvantri, VP of Research & Development, AI/ML for Digital and Signoff, Cadence on how automated processes can be useful in chip design cycle and how they directly befits the designers. We also talked about Cadence’s latest ace in the hole ‘Cerebrus’. Excerpts:
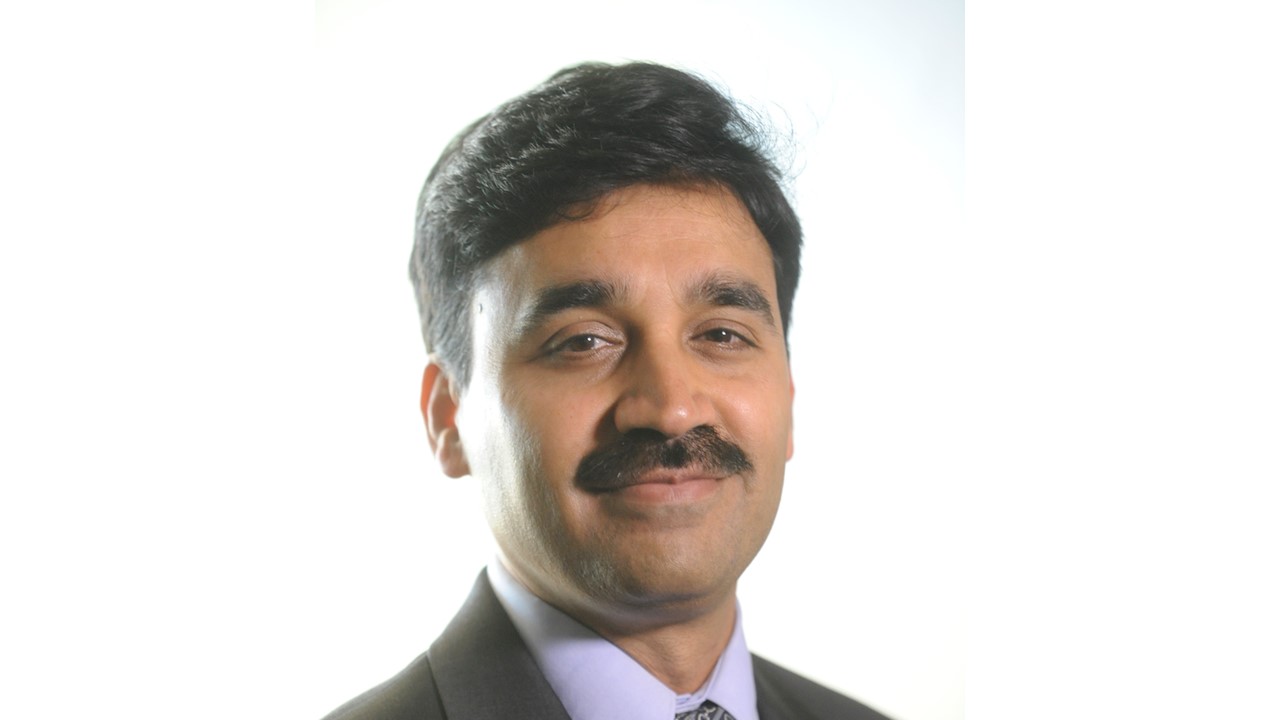
ELE Times: How does ML can provide the means to shorten the chip design cycle, creating a more integrated relationship between hardware and ML, with each fuelling advances in the other?
Cadence: To enable the semiconductor industry to continue growing, the chip design process must become more efficient. With the availability of massive, cloud-enabled, distributed computing and advancements in machine learning, the next chip design automation revolution is now possible.
ELE Times: How Cerebrus is different from other ML tools for designing as Google has also introduced ML as a tool in chip design (Reinforcement Learning Model).
Cadence: The Cadence Cerebrus Intelligent Chip Explorer utilizes both distributed computing and machine learning technologies to deliver better power, performance, and area (PPA) more quickly. Cerebrus is based on the industry-leading Cadence digital full flow. Engineering teams now are able to scale and become more productive using the Cerebrus reinforcement learning engine to meet the challenges of increasingly large and more complex system-on-chip (SoC) designs. The key differentiator here is that Cerebrus addresses machine learning across the full digital flow, rather than just one part of the flow.
ELE Times: What benefits does ‘Cerebrus’ cater to the design engineers?
Cadence: Although design technology has become much more sophisticated over the decades, the basic chip design flow has remained the same. One of today’s biggest design challenges is about a shortage of skilled design and implementation engineers. Current teams are overloaded, which impacts the ability of companies to bring new products to market. The reality is that future chips must be produced faster with more automation.
Fortunately, during the past few years, some key technologies have become available that will enable the next big leap forward in chip design productivity: Engineering teams now have access to massive compute power, either on premises or using cloud resources, and machine learning has made significant progress, and is now ready and available for electronic design automation purposes. Both these technologies have enabled the next revolution in chip design—automated, machine learning-driven flow optimization, which is where Cerebrus comes in.
By using a completely automated, machine learning-driven, RTL-to-GDS full-flow optimization technology, Cerebrus can deliver better Power, Performance and Area (PPA) results more quickly than a manually tuned flow, thereby improving engineering team productivity.
ELE Times: The process of Digital Design can be a problem-solving method considering the cognitive and strategic results it offers. Please Comment on the Statement from your perspective and in terms of the services offered by Cerebrus.
Cadence: Digital design implementation has been quite heavily dependent on the knowledge and expertise of the designers, and the quality of results vary a lot across designers or teams and the methods and processes they follow. Using the Cerebrus-based implementation flow, design companies can leverage ML technology to scale their operations to design a lot more circuits more efficiently, bringing higher productivity to the design teams.
ELE Times: What are your thoughts on The Future of ML-enhanced EDA Tools?
Cadence: ML can bring tremendous benefits to electronic design automation (EDA). ML allows EDA tools to become efficient and scalable as design size and complexity grow. We are still in the very early stages of applying ML technologies to the EDA industry and tools. Like the automotive industry is going towards self-driving, EDA tools using ML technologies will become much easier to use and “self-drive” towards the best solutions. The emphasis here is on steering the solution towards decisions or actions that are likely to produce a more favourable result (usually measured as better power, performance, and area, or PPA), where the technology quickly finds solutions that human engineers might not naturally try to explore.
Mayank Vashisht | Sub Editor | ELE Times